Vision, Goals, and Strategic Alignment
In 2015, Roundforest approached Temy with a bold idea and a challenging mission: to transform the online shopping experience by building a platform powered by intelligent data. At a time when e-commerce was becoming increasingly competitive, Roundforest aimed to simplify the decision-making process for users by delivering highly relevant product recommendations and pricing comparisons across multiple vendors.
Their vision was rooted in the power of Big Data. The platform needed to gather information on millions of products, update it in real time, and present it in a format that was both accurate and actionable. This required far more than a conventional development team—it called for a technology partner capable of handling data at scale, with robust engineering practices, and a strong understanding of end-user needs.
Temy was selected not just for its technical capability, but for its collaborative approach to long-term problem-solving.
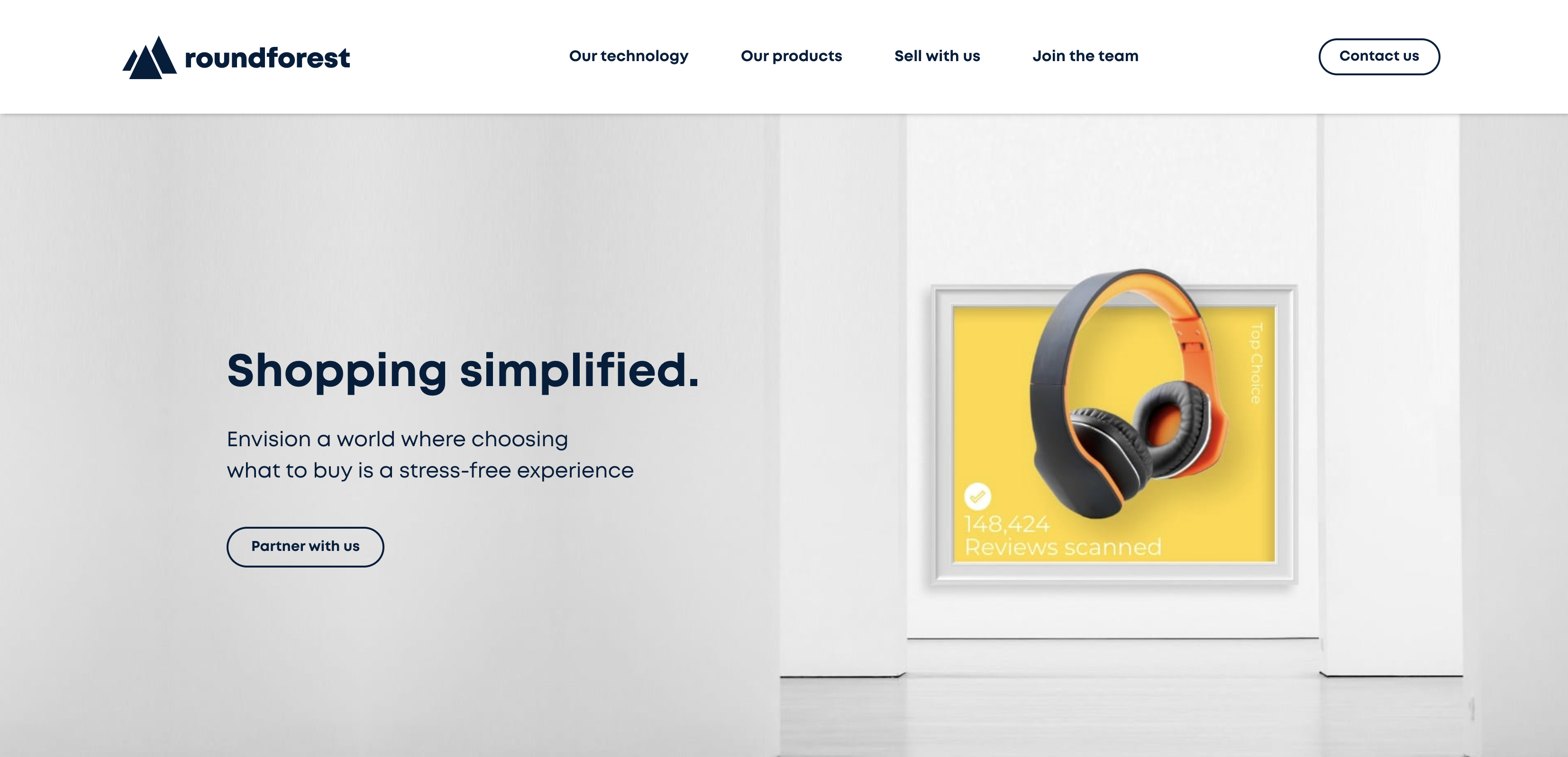
Defining the Challenge: Precision at Scale
At its core, Roundforest’s platform had to function as a highly intelligent, fully automated comparison engine—processing immense volumes of data from hundreds of retail sources. The system would extract and standardize product details, pricing, and availability, then distribute this data across a network of branded consumer sites. Moreover, every click and interaction from users had to be captured and analyzed in real time to support adaptive recommendations and performance tracking.
These demands created several complex technical challenges:
Accuracy: Even minor inconsistencies in product matching or pricing could degrade trust and lead to missed revenue opportunities.
Scalability: The data ingestion infrastructure had to grow seamlessly with the platform’s user base and inventory volume.
Actionable Insights: Analytical outputs had to be reliable, timely, and accessible to both technical and non-technical stakeholders.
Our task was to build a foundation that balanced operational efficiency with analytical sophistication, and to ensure that every component of the system—from ingestion to interface—functioned with consistency and resilience.
Crafting the Architecture: A Unified Analytical and Operational Ecosystem
Rather than rushing into development, we initiated the project with a deep discovery phase. Working closely with Roundforest’s leadership and product teams, we mapped out a full spectrum of requirements—technical, functional, and business.
This collaborative discovery led to a solution architecture designed around three core pillars:
Data Collection & Behavior Tracking: Google Analytics was deployed for real-time capture of user interactions and behavior patterns.
Data Warehousing & Historical Analysis: Amazon Redshift was selected to house large volumes of product and user data, enabling complex analytical queries with high performance.
Data Visualization & Accessibility: Metabase was integrated as a lightweight, open-source BI tool that allowed non-technical stakeholders to explore data independently.
On the backend, our team engineered a modular API infrastructure to enable multiple shopping properties to operate using a centralized intelligence engine. This allowed Roundforest to maintain consistency while delivering differentiated experiences across various consumer-facing platforms.
Engineering Delivery: Scrum Execution in a High-Stakes Environment
Our delivery process followed Scrum principles—structured but flexible. We worked in two-week sprints, conducting sprint planning, backlog grooming, demos, and retrospectives in coordination with Roundforest’s internal roadmap.
Key achievements during the development phase:
Rapid MVP Delivery: The initial minimum viable product (MVP) was developed, tested, and launched within several months, enabling Roundforest to go to market and begin validating hypotheses with real users.
Incremental Optimization: Through A/B testing and phased rollouts, we iteratively improved algorithms, user interfaces, and recommendation logic based on live user data.
QA-Driven Data Validation: Our QA specialist implemented automated tests for data accuracy, event schema validation, and synthetic data generation—ensuring analytical reliability at all times.
System Resilience & Monitoring: Performance logging, redundancy protocols, and load balancing mechanisms were implemented to support uptime and ensure scalability as the user base expanded.
Throughout the project, we prioritized responsiveness. Whenever data anomalies or unexpected behavioral patterns emerged, we were able to act quickly—troubleshooting, iterating, and deploying solutions with minimal disruption